
Research at the Theory Department
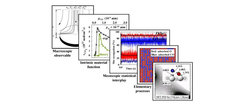
Multiscale Modeling and Data Science in Materials, Energy and Catalysis
As in many other areas of materials science, modern computational science has become a key contributor in the quest to quantitatively understand and tailor molecular-level mechanisms underlying macroscopic phenomena and functionalities in catalysis and energy applications, ultimately enabling a rational design of novel catalysts, energy suppliers and improved production strategies. Of particular relevance are hierarchical approaches that link the insights that modeling and simulation can provide across all relevant length and time scales. At the molecular level, first-principles electronic-structure calculations unravel the making and breaking of chemical bonds. At the mesoscopic scale, statistical simulations account for the interplay between all elementary processes involved in the catalytic cycle, and at the macroscopic scale continuum theories yield the effect of heat and mass transfer, ultimately scaling up to a plant or device-wide simulation. A comprehensive control of catalytic and energy processes requires combining all of these facets and thus necessitates novel methodological approaches that integrate the various levels of theory into one multiscale simulation. At present, corresponding developments are further fueled by the fusion with emerging techniques from machine learning and artificial intelligence. Modelling and simulation, and in particular powerful virtual computational screening approaches, create vasts amounts of data. Efficiently extracting the knowledge contained in this data requires optimized data representations for the chemical problems at hand (feature engineering) that then enable modern data analytics (classification, regression, network analysis). Vice versa, machine learning and especially active learning concepts are key to minimize the computationally intense generation of first-principles data required as foundation for predictive-quality (multiscale) models.
Within this context our research presently concentrates on the following focus areas:
- Energy Conversion Processes
- Heterogeneous Catalysis
- Surface Nanotechnology
- Multiscale Modeling and Data Sciences
- Multiscale Modeling Methodology
- Kinetic Monte Carlo
- Global Geometry Optimization and Crystal Structure Prediction
- Machine Learned Potentials
- Graph Theoretical Approach to Reaction Networks
- Data Mining and Computational Materials Design
Uncertainty and Inverse Problems
Computational Fluid Dynamics and Reduced Order Reactor Modelling and Simulation
Automatic Process Exploration
- First-Principles Method Development
Heat and Charge Transport
Time-Dependent Density Functional Theory
Machine-Learning Enhanced Semiempirical Electronic Structure
Further information about our research approach can be found in our following reviews on
Exploring catalytic reaction networks with machine learning (2023)
Human- and machine-centered design of molecules and materials (2022)
Implicit solvation methods for catalysis at electrified interfaces (2022)
Adsorption enthalpies for catalysis modeling through machine-learned descriptors (2021)
A practical guide to surface kinetic Monte Carlo simulations (2019)
Recent developments in computational surface catalysis (2018)
Ab initio thermodynamics and first-principles microkinetics (2016)
First-principles kinetic modeling in heterogeneous catalysis (2012)
For further information don't hesitate to contact us, or browse through our recent publications.