Data-Enabled Materials Structure-Property-Synthesizability Predictions
- TH Department Online Seminar
- Date: Jan 20, 2022
- Time: 10:00 AM - 11:00 AM (Local Time Germany)
- Speaker: Professor Yousung Jung
- Department of Chemical and Biomolecular Engineering, KAIST, Daejeon, South Korea
- Location: https://zoom.us/j/93668499051?pwd=dWVjZ2pIaGxINjgwTG42ZTkwWnpTdz09
- Room: Meeting ID: 936 6849 9051 | Passcode: 073098
- Host: TH Department
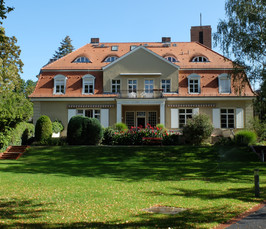
In forward mapping, one aims to predict the materials properties using their structures, encoded in various ways such as simple attributes of constituent atoms, compositions, or structures in graph forms, while in inverse mapping, one defines the desired property first and attempts to find the materials with the target property using an inverse algorithm. In this talk, I will talk about some of our recent developments in machine learning methods for materials property predictions [1-3] and inverse design [4-6], and also describe methods to predict the synthesizability of inorganic crystals and molecules based on their structures or chemical compositions [7,8].
References
[1] “A Local Environment Descriptor for Machine-Learned Density Functional Theory at the Generalized Gradient Approximation Level”, Ji & Jung, J Chem Phys 148, 241742-241751 (2018)
[2] “Practical Deep-Learning Representation for Fast Heterogeneous Catalyst Screening”, Gu, Noh, Kim, Back, Ulissi & Jung, J Phys Chem Lett 11, 3185-3191 (2020)
[3] “Autobifunctional Mechanism of Jagged Pt Nanowires for Hydrogen Evolution Kinetics via End-to-End Simulation”, Gu, Lim, Wan, Cheng, Pu, Kim, Noh, Choi, Kim, Goddard, Duan & Jung, J Am Chem Soc 143, 5355-5363 (2021)
[4] “Generative Adversarial Networks for Crystal Structure Prediction”, Kim, Noh, Aspuru-Guzik & Jung, ACS Cent Sci 6, 1412-1420 (2020)
[5] “Inverse Design of Solid State Materials via a Continuous Representation”, Noh, Kim, Stein, Sanchez-Lengeling, Gregoire, Aspuru-Guzik & Jung, Matter 1, 1370-1384 (2019)
[6] “Machine-Enabled Inverse Design of Inorganic Solid Materials: Promises and Challenges”, Noh, Gu, Kim & Jung, Chem Sci 11, 4871-4881 (2020)
[7] “Structure-based Synthesizability Prediction of Crystals using Partially Supervised Learning”, Jang, Gu, Noh, Kim & Jung, J Am Chem Soc 142, 18836-18843 (2020)
[8] “Deep Retrosynthetic Reaction Prediction using Local Reactivity and Global Attention”, Chen & Jung, JACS Au 1, 1612-1620 (2021)