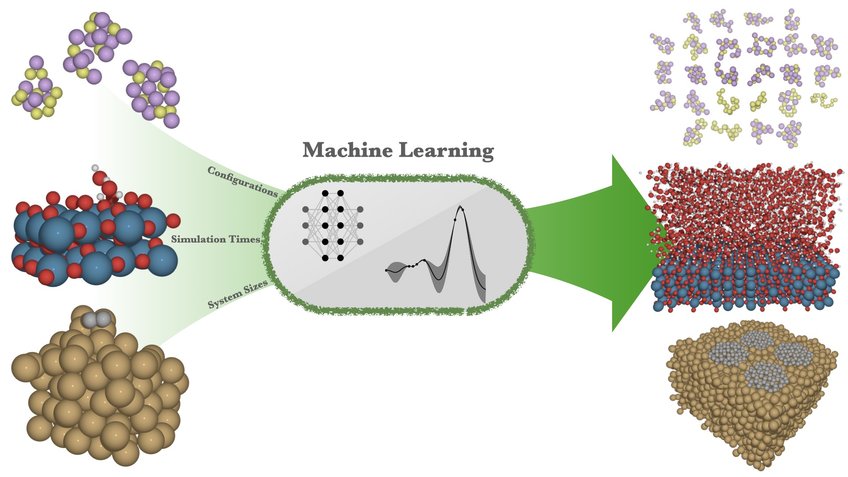
Interatomic machine learning potentials for energy materials
Heenen Group
In our group we develop machine-learned interatomic potentials for materials that are at the heart of energy conversion processes in battery technology and catalysis.
Battery systems and (electro)catalysis applications are key technologies for the efficient storage of renewable energy. Many of the major challenges still limiting these technologies arise at interfaces which are inherently complex and therefore often poorly understood. Atomistic simulations which could potentially rationalize these interfaces require a high accuracy and exhaustive sampling. Here, direct first-principles (e.g. Density Functional Theory, DFT) or empirical force field calculations are often severely limited due to prohibitive computational cost or insufficient accuracy, respectively. In contrast, machine learned interatomic potentials (MLIP) offer an alternative route forward as they show great promise of providing both sufficient accuracy and efficiency - thus filling an important application gap.
In our group, we develop strategies to train reliable MLIP which can capture complex chemical spaces and reactive chemistry. The target MLIP are intentionally designed to be as general as possible and go beyond single-purpose acceleration schemes where a MLIP is trained to replace e.g. DFT in a specific task. A major part of our methodological work includes active learning and uncertainty quantification as well as the incorporation of long-range interactions. The development of general-purpose workflows and concepts to "automatically" obtain potentials with limited user input is a long-term goal, which we persue by collaboratively co-developing the libatoms/workflow code. We develop and adapt our efforts alongside the investigation of technologically relevant systems that would be otherwise inaccessible (i.e. with DFT), such as Li-Sulfur battery chemistry, deactivation processes of electrocatalytic catalysts and graphene synthesis.
