Accurate and Transferable DFT: Machine-Learned aPBE0 and Physics-Based XDM in FHI-Aims
- NOMAD Laboratory
- Date: Apr 15, 2025
- Time: 10:15 AM (Local Time Germany)
- Speaker: Dr. Alastair Price
- Department of Chemistry, Acceleration Consortium, University of Toronto, Canada
- Location: Building T
- Room: 0.18/0.19
- Host: NOMAD Laboratory
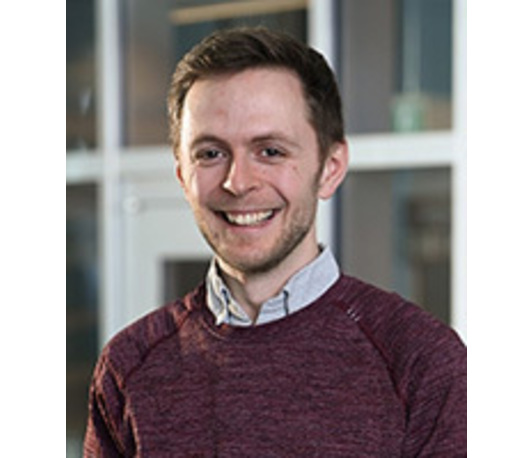
Abstract:
I present the implementation and application of the accurate,
machine-learned aPBE0 functional and the exchange-hole dipole moment
(XDM) dispersion correction within the FHI-aims code. The aPBE0
functional, trained on benchmark molecular data, effectively
mitigates delocalization error while retaining the computational cost
of hybrid DFT. Its non-empirical design yields reliable energetics
across a broad chemical space. Combined with XDM, which I have extended
to solids and molecular crystals within FHI-aims,
the approach delivers state-of-the-art accuracy in cohesive and lattice
energy predictions. This combination offers a transferable, physically
grounded framework for tackling noncovalent interactions in condensed
phase systems.
Bio:
Alastair J. A. Price is a postdoctoral fellow at the University of Toronto and the Acceleration Consortium, working with Professors O. Anatole von Lilienfeld and Alán Aspuru-Guzik. He completed his PhD under Professor Erin R. Johnson at Dalhousie University, where he developed a new implementation of the XDM dispersion model. His research focuses on advancing accurate and transferable density functionals and dispersion corrections, including the machine-learned aPBE0 functional and XDM for solids and molecular crystals within FHI-aims.