Uncertainty-Aware Exploration of Atomic Energy Landscapes
- TH Department Seminar
- Date: Apr 24, 2025
- Time: 02:00 PM (Local Time Germany)
- Speaker: Dr. Thomas D Swinburne
- Centre Interdisciplinaire de Nanoscience de Marseille, Campus de Luminy, Aix-Marseille Université, France
- Location: https://zoom.us/j/94580966994?pwd=D0Pg8jTSOFnR3PXgiqhr2dFmPS9zpP.1
- Room: Meeting ID: 945 8096 6994 | Passcode: 136076
- Host: TH Department
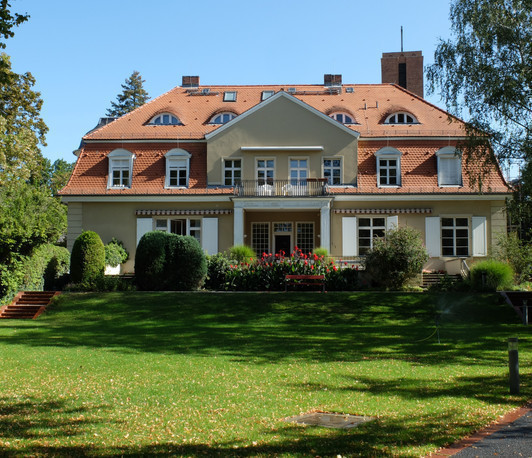
Atomic simulations allow fantastic insight for chemistry and materials science, but in silico data has many uncertainties which complicate interpretation and prediction. I will discuss recent Bayesian methods to quantify two important sources of uncertainty: those arising from imperfect models for atomic interaction[1,2] and incomplete sampling of the atomic energy landscape[3].
To quantify interatomic potential uncertainty we must revisit Bayesian regression, as the expected loss provably ignores model-form error. I will present a solution which has minimal overhead yet provides robust max/min bounds for both linear and foundational machine learning potentials[4]. To quantify incomplete sampling uncertainty we can leverage theoretical knowledge of kinetics to derive a likelihood for observed sampling data, enabling robust bounding of model predictions and optimal autonomous management of massively parallel sampling tasks. Autonomy is essential to fully harness parallel computational resources, allowing exploration of complex energy landscapes in a few hours of walltime[5,6].
[1] TDS and D Perez, Mach. Learn. Sci & Tech 2025
[2] Maliyov, Grigorev, TDS, NPJ Comp. Mat. 2025
[3] TDS and D Perez, NPJ Comp. Mat. 2020
[4] github.com/tomswinburne/POPS-Regression
[5] Goryaeva et al., Nat. Comm. 2023
[6] github.com/tomswinburne/tammber.git
[2] Maliyov, Grigorev, TDS, NPJ Comp. Mat. 2025
[3] TDS and D Perez, NPJ Comp. Mat. 2020
[4] github.com/tomswinburne/POPS-Regression
[5] Goryaeva et al., Nat. Comm. 2023
[6] github.com/tomswinburne/tammber.git