Molecules and Materials in Conversation: Encoding and Decoding Chemistry with Language Models
CatLab Lectures 2024/25
- Date: Nov 15, 2024
- Time: 10:30 AM - 12:00 PM (Local Time Germany)
- Speaker: Dr. Kevin Jablonka
- Helmholtz Institute for Polymers in Energy Applications
- Location: Building M, Richard-Willstätter-Haus, Faradayweg 10, 14195 Berlin
- Room: seminar room, 1st floor
- Host: HZB and FHI
- Contact: trunschk@fhi-berlin.mpg.de
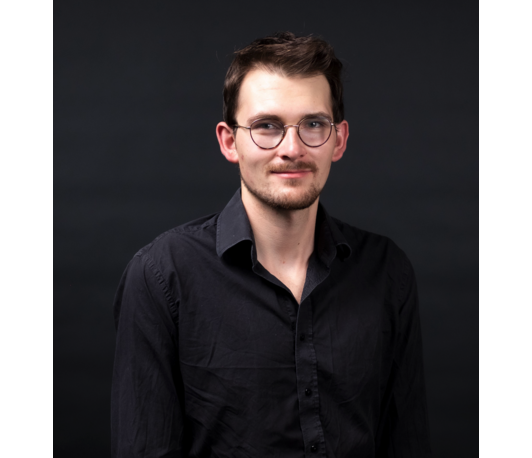
The field of chemical sciences has seen significant advancements with the use of data-driven techniques, particularly with large datasets structured in tabular form. However, collecting data in this format is often challenging in practical chemistry, and text-based records are more commonly used.
Using text data in traditional machine-learning approaches is also difficult. Recent
developments in applying large language models (LLMs) to chemistry have
shown promise in overcoming this challenge. LLMs can convert
unstructured text data into structured form and can even directly solve
predictive tasks in chemistry. In my talk, I will present the impressive
results of using LLMs, showcasing how they can autonomously utilize
tools and leverage structured data and “fuzzy” inductive biases. To
enable the training of a chemical-specific large language model, we
have curated a new dataset along with a comprehensive toolset to utilize
datasets from knowledge graphs, preprints, and unlabeled molecules. To
evaluate frontier models trained on such a dataset, we specifically
designed a benchmark to evaluate the chemical knowledge and reasoning
abilities. I will present the latest results, demonstrating the
potential of LLMs in advancing chemical research.