Across Molecular Timescales: Path Reweighting, Markov State Models and Machine Learning
- TH Department Seminar
- Date: Jul 18, 2024
- Time: 02:00 PM (Local Time Germany)
- Speaker: Prof. Bettina G. Keller
- Freie Universität Berlin, Institute for Chemistry and Biochemistry, Physical & Theoretical Chemistry, Berlin, Germany
- Location: https://zoom.us/j/95003933280?pwd=Bl67dKB3rE7fS7WSaWn2agau2JaSy3.1
- Room: Meeting ID: 950 0393 3280 | Passcode: 100587
- Host: TH Department
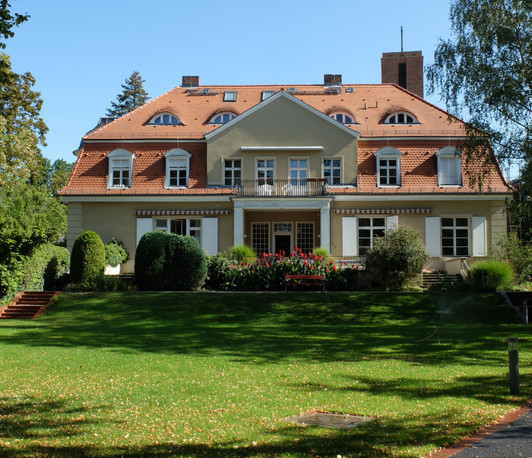
With machine-learnt molecular potential energy functions, most notably neural-network potentials, one can now sample chemical reactions and the influence of the environment on these reactions, as well as processes at high temperature and pressure at which the approximations of empirical molecular potentials break down. Because these processes are associated with extremely high free energy barriers, often 2 to 3 orders of magnitude higher than thermal energy, direct simulations of these processes is numerically inefficient, and one instead often uses enhanced sampling simulations on biased potential energy surfaces. To put it succintly: the processes for which neural-network potential are designed prohibit direct sampling of these potentials. Whether we will be able to harvest the full benefit of modern molecular potentials, critically depends on the accuracy and the usability of the dynamical reweighting method, which recover the unbiased dynamics.
I will discuss path reweighting, which unbiases molecular dynamics by explicitly calculating the path probability density in the original and biased potential. This method is exact in the sense that it does not make any assumption in the underlying dynamics, but instead uses the actual dynamics of the MD integrator for underdamped Langevin dynamics. However, the variance of the estimator drastically increases with path length. By combining path reweighting with Markov state models, which operator on a short lag time, one can control this variance and obtain precise models of the molecular kinetics. I will present recent developments in path reweighting. I will also discuss how the technique can be connected with ML methods for improving molecular potentials and optimizing reaction coordinates.
Joana-Lysiane Schäfer, Bettina G. Keller. "Implementation of Girsanov Reweighting in OpenMM and Deeptime." J. Phys. Chem. B (2024) https://doi.org/10.1021/acs.jpcb.4c01702
Stefanie Kieninger, Bettina G. Keller. "Path probability ratios for Langevin dynamics—Exact and approximate." J. Chem. Phys. (2021) https://doi.org/10.1063/5.0038408