Machine Learning (ML) for Simulating Complex Energy Materials with Non-Crystalline Structures
- TH Department Online Seminar
- Date: Jun 23, 2022
- Time: 02:00 PM (Local Time Germany)
- Speaker: Prof. Nongnuch Artrith
- Materials Chemistry and Catalysis, Debye Institute for Nanomaterials Science, Utrecht University, The Netherlands
- Location: https://zoom.us/j/94714507046?pwd=ak16cG9leDE3cnMxZ1BCeXhsZ3BnZz09
- Room: Meeting ID: 947 1450 7046 | Passcode: 535637
- Host: TH Department
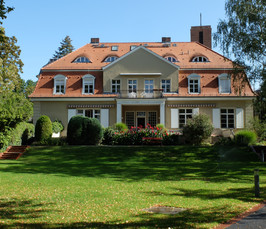
Here, I will discuss the methodology and applications to amorphous battery materials. We constructed the phase diagram of amorphous LiSi alloys, prospective anode materials for lithium-ion batteries. And we mapped the composition and structure space of amorphous LiPON and LPS solid electrolytes. The thermodynamic stability and ionic conductivity of the non-crystalline phases was correlated with local structural motifs, leading to the identification of structure-composition-conductivity relationships that can be used for materials optimization and design. Further, I will show how large computational and small experimental data sets can be integrated for the ML-guided discovery of catalyst materials. These examples show that the combination of first-principles calculations and ML models is a useful tool for the modelling of complex energy materials and for materials discovery. All data and models are made publicly available. To promote Open Science, we also formulated guidelines for the publication of ML models for chemistry that aim at transparency and reproducibility.
Artrith, Urban, Ceder, Phys. Rev. B 96 (2017) 014112; J. Chem. Phys. 148 (2018) 241711; Lacivita, Artrith, Ceder, Chem. Mater. 30, (2018) 7077-7090; Guo, Wang, Urban, Artrith, arXiv:2201.11203, 2022; Artrith, Chen, et al, ACS Catal. 10 (2020) 9438-9444; Applied Catalysis B: Environmental 312 (2022) 121380.