Machine Learning at the Atomic Scale: From Structural Representations to Chemical Insights
- TH Department Online Seminar
- Date: May 6, 2022
- Time: 02:00 PM (Local Time Germany)
- Speaker: Professor Michele Ceriotti
- École Polytechnique Fédérale de Lausanne, Lausanne, Switzerland
- Location: https://zoom.us/j/95871552113?pwd=Ti8rZTdIRzlHSFpWQ2xBZVJOc3lQUT09
- Room: Meeting ID: 958 7155 2113 | Passcode: 889419
- Host: TH Department
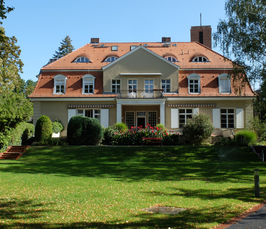
In particular, statistical regression techniques have become very
fashionable as a tool to predict the properties of systems at the atomic
scale, sidestepping much of the computational cost of accurate quantum
chemical calculations, and making it possible to perform simulations
that require thorough statistical sampling without compromising on the
accuracy of the electronic structure model.
I will show that data-driven modelling can be rooted in a mathematically
rigorous and physically-motivated symmetry-adapted framework, and discuss the benefits of taking a well-principled approach.
I will present several examples demonstrating how the combination of
machine-learning and atomistic simulations can offer useful insights on
the behavior of complex systems, and discuss the challenges towards an
integrated modeling framework in which physics-driven and data-driven
steps can be combined to improve the accuracy, the computational
efficiency and the transferability of predictions, from interatomic
potentials to electronic-structure properties.