Machine-Learning Potentials: The Accurate, the Fast, and the Applied
- TH Department Online Seminar
- Date: Mar 24, 2022
- Time: 02:00 PM (Local Time Germany)
- Speaker: Dr. Matthias Rupp
- Universität Konstanz, Computer Science, Bioinformatics and Information Mining, Konstanz, DE
- Location: https://zoom.us/j/94781924316?pwd=Nk1MalFiSmJIeit4RCs5SlpjcHVTUT09
- Room: Meeting ID: 947 8192 4316 | Passcode: 015558
- Host: TH Department
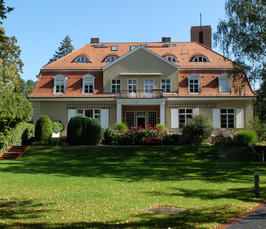
These are essential for physics, chemistry, and materials science, but severely limited
by their computational cost. Machine-learning potentials (MLPs) are data-driven surrogate models that accurately
interpolate between a few selected reference calculations at orders of magnitude lower computational cost, enabling
accurate dynamics simulations at otherwise inaccessible system sizes and time scales. I will present "ultra-fast
potentials" based on splines, prediction of thermal transport in materials via the Green-Kubo formalism and deep network
MLPs, and outline first steps towards MLPs based on quantum Monte Carlo calculations. In doing so, I will discuss the
role of domain knowledge and trade-offs involved in the design of MLPs.