Towards ex-machina computations of transport and transformations in complex materials
- Online Seminar of the NOMAD Laboratory
- Date: Jun 8, 2021
- Time: 04:00 PM (Local Time Germany)
- Speaker: Boris Kozinsky
- Associate Professor of Computational Materials Science Harvard School of Engineering and Applied Sciences
- Location: Join the webinar: https://us02web.zoom.us/j/81226137542?pwd=Qm9NRWY3TlZ4RFVnVjNhZklHTm82dz09
- Room: Meeting ID: 812 2613 7542 I Password: NOMAD
- Host: NOMAD Laboratory
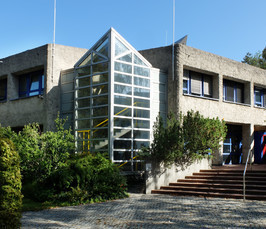
Harnessing
the accuracy of quantum mechanics to
design complex materials requires a series
of approximations to reach the desired
length and time scales. In the context of
thermoelectric materials, new automatable
computational methods for describing
electron-phonon dynamics enabled us to
discover new leading-efficiency alloy
compositions. In the context of batteries,
understanding of ionic transport
mechanisms is important for enabling
next-generation solid-state electrolytes.
To advance the ability to capture
complexity of ionic, thermal and reaction
dynamics, we are pursuing the paradigm of
“ex-machina†computations where
data-driven approximations are
automatically developed using machine
learning algorithms. To accelerate
molecular dynamics calculations, we
developed the Neural equivariant
interatomic potential model (NequIP) based
on tensor-valued symmetry-preserving
neural network architectures and used them
to achieve state-of-the-art accuracy and
training efficiency for molecules,
liquids, heterogeneous catalysts, and
ionic conductors. In order to enable
autonomous selection of the training set
for reactive systems, we developed the
FLARE adaptive closed-loop algorithm that
automatically constructs accurate
non-parametric interatomic force fields
on-the-fly from a molecular dynamics
simulation. We demonstrate the performance
of ML-accelerated MD simulations by
studying 2D-to-3D transformations of
quantum materials, shape memory effect in
alloys and thermal transport in
semiconductors.