Interpretable Artificial Intelligence for the not-so-big Data of Materials Science
A Joint Seminar of the NOMAD Laboratory and of the Ma group
- Online Seminar of the NOMAD Laboratory
- Date: Jan 25, 2021
- Time: 10:15 AM (Local Time Germany)
- Speaker: Dr. Luca Ghiringhelli
- NOMAD Laboratory of the Fritz Haber Insitute of the Max Planck Society
- Location: Join the webinar: https://us02web.zoom.us/j/82167216990?pwd=bXUxeTVqRzdZeDJJRWZuUnFIRmtPdz09
- Room: Webinar ID: 821 6721 6990 I Password: NOMAD
- Host: NOMAD Laboratory
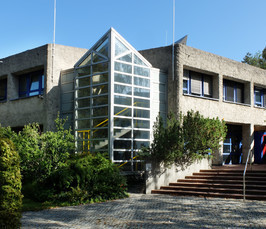
A desirable strategy would be to start from a consistent body of experimental or theoretical data, and by means of artificial-intelligence (AI) methods, to identify yet unseen patterns or structures in the data, and consequentially predictive (data-driven) models. This leads to the identification of maps (or charts) of materials where different regions correspond to materials with different properties. The main challenge on building such maps is to find the appropriate descriptive parameters (called descriptors) that define these regions of interest.
Here, I present novel methods for the AI-aided identification of descriptors and materials maps, tailored to work (also) with "small-data", and applied to important materials-science challenges such as the prediction of stability of perovskite materials, of novel topological insulators, and more. I focus on the (verified) predictive power of the learned maps, which goes beyond the mere interpolation of more "traditional" AI approaches, and analyze current and future challenges.