Smart Sampling for Chemical Property Landscapes with BOSS
A Seminar of the NOMAD Laboratory
- Online Seminar of the NOMAD Laboratory
- Date: Jan 14, 2021
- Time: 01:15 PM (Local Time Germany)
- Speaker: Milica Todorović
- Aalto University, Finland
- Location: https://us02web.zoom.us/j/83575269594?pwd=WFpLUjR2bDFESzdlZmlDVlN4eEwzQT09
- Room: Webinar ID: 835 7526 9594 | Password: NOMAD
- Host: NOMAD Laboratory
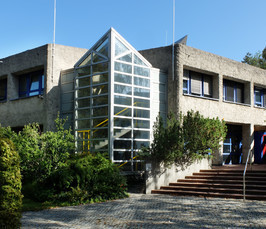
We applied this active learning scheme to study 1) molecular adsorption at the organic/inorganic interfaces and 2) molecular conformers. For 1), we studied camphor deposited on Cu(111) and identified 8 unique stable adsorbates [2]. By comparing them to atomic force microscopy (AFM) images, we were able to recognize 3 different structures of chemisorbed camphor in experiments [3]. For 2), we applied BOSS to conformer search of several amino acid molecules. We successfully recovered more than 10 experimentally and theoretically determined conformer structures in less than 10% computational cost of the current fastest method [4]. With a recent batch implementation for active learning, BOSS can make use of exascale computing resources to solve large-scale structural problems without sacrificing quantum-mechanical accuracy.
References:
[1] M. Todorović, M. U. Gutmann, J. Corander and P. Rinke, ‘Efficient Bayesian Inference of Atomistic Structure in Complex Functional Materials’, npj Comput. Mater., 5, 35 (2019)
[2] J. Järvi, P. Rinke and M. Todorović, ‘Detecting stable adsorbates of (1S)-camphor on Cu(111) with Bayesian optimization’ Beilstein J. Nanotechnol. 11, 1577-1589 (2020)
[3] J. Järvi, B. Aldritt, O. Krejčí, M. Todorović, P. Liljeroth and P. Rinke, ‘Integrating Bayesian Inference with Scanning Probe Experiments for Robust Identification of Surface Adsorbate Configurations’, under consideration at Adv. Funct. Mater., doi:10.21203/rs.3.rs-50783/v1, (2020)
[4] L. Fang, E. Makkonen, M. M. Todorović, P. Rinke, X. Chen, ’Efficient Cysteine Conformer Search with Bayesian Optimization’, accepted at JCTC, arXiv:2006.15006 (2020).