Datasets, Workflows, Models and Active Learning to Accelerate Catalyst Discovery
- TH Department Online Seminar
- Date: Dec 3, 2020
- Time: 02:00 PM - 03:00 PM (Local Time Germany)
- Speaker: Prof. Dr. Zachary W. Ulissi
- Department of Chemical Engineering, Carnegie Mellon University, Pittsburgh, PA, USA
- Location: Join zoom meeting: https://tum-conf.zoom.us/j/95962763450 | Webinar ID: 959 6276 3450 | Passcode: 212179
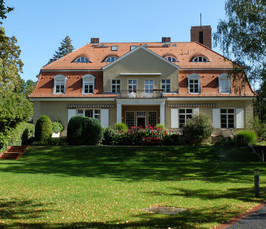
However, there are several large challenges that remain: to
date, models have had trouble generalizing to new materials or reaction
intermediates and applying these methods requires significant researcher
training. I will review and discuss methods in my lab for
high-throughput catalyst screening and on-line discovery of interesting
materials, resulting in an optimized Cu-Al catalyst for CO2-to-ethylene
conversion. I will then introduce the Open Catalyst Project and the Open
Catalyst 2020 dataset, a collaborative project to span surface
composition, structure, and chemistry and enable a new generation of
deep machine learning models for catalysis, with initial results for
state-of-the-art deep graph convolutional models. Finally, I will
discuss on-going work to develop small ML models to accelerate routine
calculations without requiring expert intervention.