AI Approach for Heterogeneous Catalysis
A tailored artificial intelligence approach can be applied to model catalysis. It highlights the underlying physicochemical processes, and accelerates catalyst design.
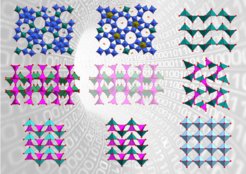
Artificial intelligence (AI) accepts that there are relationships or correlations that cannot be expressed in terms of a closed mathematical form or an easy-to-do numerical simulation. For the function of materials, for example, catalysis, AI may well capture the behavior better than the theory of the past. However, currently the flexibility of AI comes together with a lack of interpretability, and AI can only predict aspects that were included in the training.
Researchers from the Novel-Materials Discovery (NOMAD) Laboratory at the FHI together with researchers from the FHI Inorganic Chemistry Department and the BasCat UniCat BASF JointLab at TU Berlin have now proposed an interpretable AI approach for modelling heterogeneous catalysis. It starts with the catalyst synthesis, detailed characterization and testing according to systematic experimental procedures, which generate consistent and well-characterized data, so-called "clean data". Then, symbolic regression is applied for the identification of the key physicochemical descriptive parameters that are correlated, in a possibly nonlinear way, with the catalytic performance. These parameters reflect the processes that trigger, facilitate, or hinder the reactivity and, in analogy to genes in biology, they might be called "materials genes of heterogeneous catalysis".
This approach, demonstrated for the selective oxidation of propane catalyzed by nine vanadium-based materials, will accelerate the discovery of improved or novel materials while also enhancing physical understanding.